Advertisement
Speaking My Language
Translation Technology Is Getting Better. What Does That Mean For The Future?
Resume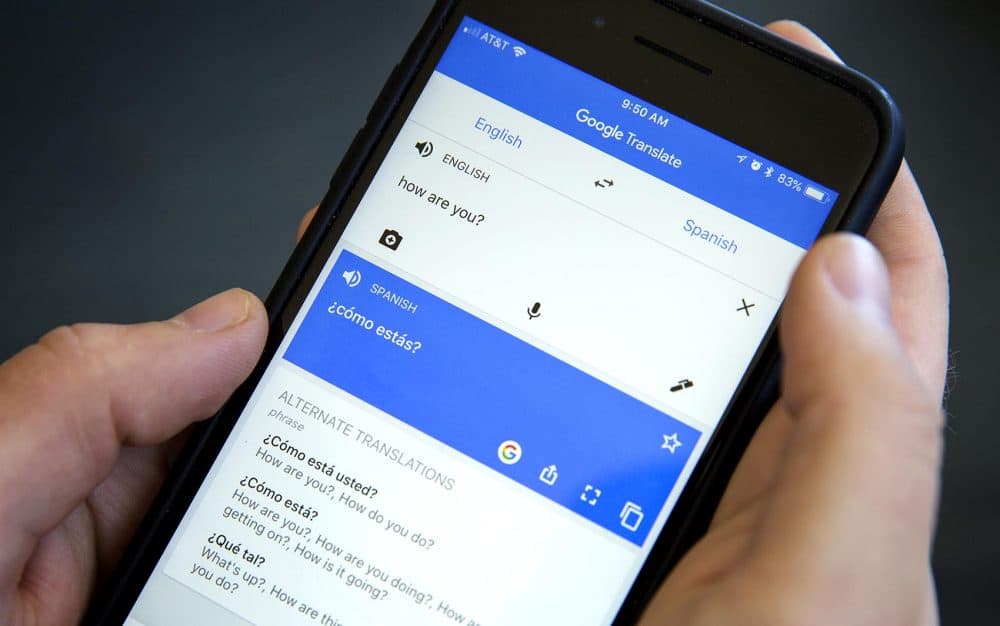
Tools and apps like Google Translate are getting better and better at translating one language into another.
Alexander Waibel, professor of computer science at Carnegie Mellon University's Language Technologies Institute (@LTIatCMU), tells Here & Now's Jeremy Hobson how translation technology works, where there's still room to improve and what could be in store in the decades to come.
Interview Highlights
On how technology like Google Translate or Microsoft's Translator works
"Over the years I think there's been a big trend on translation to go increasingly from rule-based, knowledge-based methods to learning methods. Systems have now really achieved a phenomenally good accuracy, and so I think, within our lifetime I'm fairly sure that we'll reach — if we haven't already done so — human-level performance, and/or exceeding it.
"The current technology that really has taken the community by storm is of course neural machine translation. It has essentially taken the entire community only one year to adopt it, largely because of very, very good performance increases over statistical translation. And before that, statistical translation took over from rule-based methods, and with each one of these jumps, I would say the main benefits were that more things were essentially done by learning, as opposed to making modeling assumptions or rules."
"Within our lifetime I'm fairly sure that we'll reach — if we haven't already done so — human-level performance, and/or exceeding it."
Alexander Waibel, on how quickly language technology is improving
On what terms like neural machine translation and statistical translation mean
"First of all, when people do rule-based translation, they try to do everything by linguistic rules. And what you have to do then is create rules that explain the context of a sentence well enough so that you can pick the correct word in the output language. Let's say I use the word 'bank,' then are we talking about the river bank? Or are we talking about the financial institution? The answer is it really depends on the context. And in the past, people have done this by rules, by taking complex syntactic parsers, by using large dictionaries and ontologies to explain the semantics of the world. But as you and I know, that of course always leads to these jokes and these funny translations where the system simply doesn't know about some of the semantic differences that we have.
"The biggest problem in machine translation always is the ambiguity. We just don't know what a word means — if you say, 'If the baby doesn't like the milk, boil it,' do you mean boiling the milk, or boiling the baby? We all know that we mean boiling the milk, but that's because we know that we don't boil babies, right? But if you now translate it to German, then you need to know which one you meant, because it could then refer to a baby or to milk. So we really have to resolve these issues, and doing this by rule was essentially a daunting task, and people eventually gave up because you can't simply describe all the facts that we learn during a lifetime by programming rules."
Advertisement
"I think what automatic translation does for us as humans is really open doors."
Alexander Waibel
On what kind of translation came next
"The next level really in the history was to go to statistical machine translation, where these things were eased up by essentially counting. The technology's pretty easy to explain because you create effectively a dictionary, and the dictionary has multiple translations, so 'bank' would be the financial institution, and you have another word for the river bank, and then you assign the probability. How likely is it that I'm talking about a river bank or a financial institution? Just the word by itself. And then the statistical models would then also consider the context, and that too you can simply derive from large amounts of data. The Googles of the world obviously have a lot of data, and so by taking a ton of text data, you count how often is 'bank' translated as a financial institution, when you had as a context 'Deutsche,' Deutsche Bank, and do you talk about the river bank, if I say the word 'Mississippi' and the river bank."
On progressing to neural machine translation
"The neural machine translation effectively, you would say, is also a statistical learning. However, it does this with multiple layers of abstraction. So that's where the word 'deep' comes from, because you have several layers of neurons that build on top of each other. And what that gives you is a way of abstracting at various levels about language, about speech, about vision, without us actually explicitly telling it."
On if this technology will eventually make it so that people don't have to learn other languages
"We get that question a lot, but actually I happen to be a bit contrarian in the sense that I think it's going to be the opposite. Because I think what automatic translation does for us as humans is really open doors. So we wind up actually traveling to countries or meeting people that we would have otherwise never met or never interacted with.
"So I think having technology as a door-opener I feel will actually help us to make more contact with people who speak other languages, simply because it is nice, it opens our world more and we start understanding each other more, and that's of course what it's all about."
This article was originally published on July 19, 2018.
This segment aired on July 19, 2018.